In 2022, the visual generative artificial intelligence (AI) tools Midjourney and DALL-E hit the scene, both letting creators input text prompts to bring wild conjurings to life as realistic renderings. According to Stanislas Chaillou, author of “Artificial Intelligence and Architecture,” AI is the latest major development in architectural technology. Although it’s easy to get swept up in the glitzy generative side, designers are finding many more ways that AI can expand creativity while saving time, money and brainpower for more rewarding tasks.
In London, for example, the Applied Research and Development Group (ARD) at Foster + Partners began applying AI and its offshoot machine learning (ML) in 2017. The group used it for models ranging from design-assist, surrogates, knowledge dissemination, business insight—and, yes, its own take on diffusion models that generates images from natural language. Los Angeles-based Verse Design tapped AI to meet aesthetic and performance criteria for a structure that recently won a 2023 A&D Museum Design Award.
But implementing AI doesn’t come without obstacles—including questions about protecting intellectual property (IP), training with appropriate datasets and defining creativity when it seems to lie with the designer of the AI script.
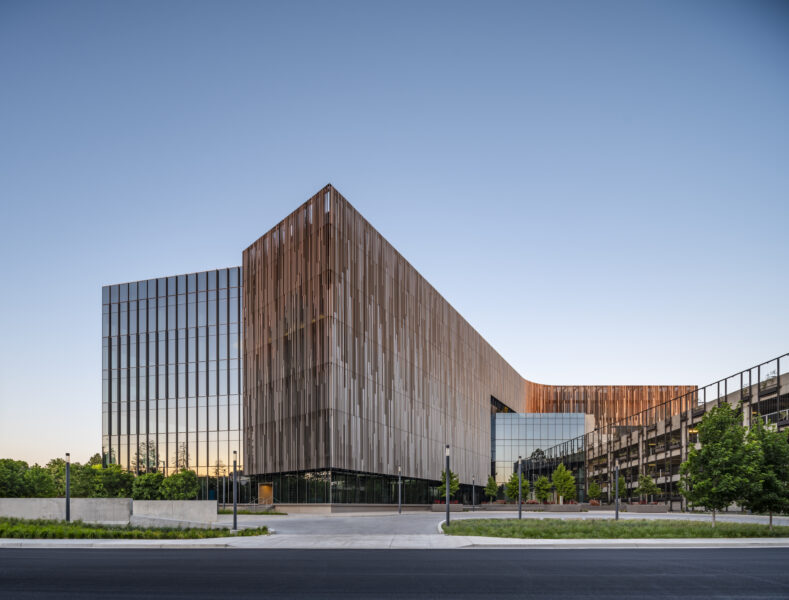
AI design assistance arrives
One ARD Group study involved laminates that self-deform when subject to temperature, light or humidity. The materials would enable a façade that responds differently depending on conditions to provide shading, prevent overheating or increase privacy. But to simulate the laminates’ nonlinear and unpredictable response, the group turned to ML.
“We used ML to predict how a passively actuated material would react to variable temperature changes,” said Martha Tsigkari, senior partner. “With the help of our bespoke distributed computing and optimization system, Hydra, we ran thousands of simulations to understand how thermoactivated laminates behave under varied heat conditions. We then used that data to train a deep neural network to tell us what the laminate layering should be, given a particular deformation that we required.”
Predicting material deformation was just one application. To help automate mundane tasks and turbo-power productivity, the ARD Group is working on many more ideas around AI-powered design assist tools.
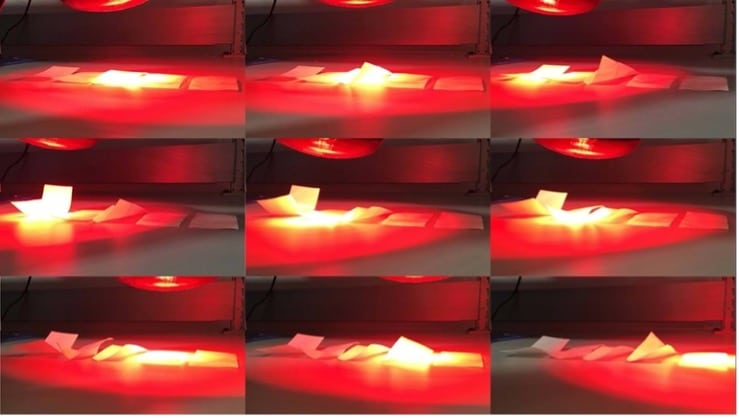
Verse Design faced similar performance constraints when designing the façade of Thirty75 Tech. The designers needed to find the optimal pattern of louvers to mitigate heat gain and meet California’s Title 24 energy efficiency standards.
“The final geometries were generated parametrically with real-time simulation data,” Tang explained. “The geometries were fed back to the energy model to find and confirm the most energy-efficient combination of louver variations that met the intent of the visual expression and performance objectives.”
Extraordinary content delivered faster
Foster + Partners has also used surrogate models to replace slow analytical processes—and keep costs in check—when exploring the impact of changing design variables. These ML models train on huge datasets to deliver a prediction that is sufficiently exact and, most critically, available in real time. In early design stages, the surrogate model lets designers balance accuracy with the ability to make sound decisions sooner.
Curious why 3 million AECO professionals worldwide use Bluebeam to finish projects faster?


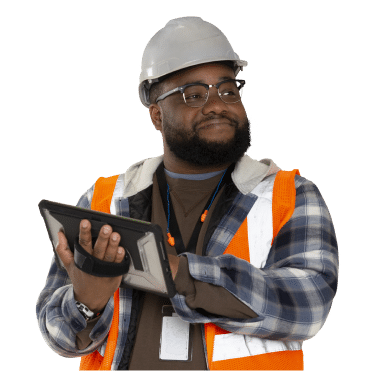
Foster + Partners’ in-house application programming interface (API) lets clients connect from digital content creation tools. With these plug-ins, users can run predictions directly. The interface also lets designers deploy diffusion models like Midjourney to stir imaginings.
“The capability of these transformers-based models to describe images, understand their context and make suggestions based on it has moved the discussion from image manipulation to natural language processing for content creation,” Tsigkari said.
Intellectual property creates a conundrum
Some creators express concern about losing control of intellectual property when feeding their own assets into AI apps. For instance, class-action lawsuits against software providers contest use of copyrighted images to train systems. Tsigkari stressed the need to understand security and IP considerations and read terms and conditions before using any software. But the challenges go beyond IP.
“It is not only the fuzzy boundaries around IP that are argued,” she said. “The lack of robust legal frameworks to deal with AI and ringfence how data may be used are going to challenge how these technologies are implemented.”
Tang doesn’t have the same concerns about IP. “As Voltaire said, ‘Originality is nothing but judicious imitation,’” he commented. “The idea is not to mindlessly copy but to critically apply the technology as a tool with generative capabilities. It requires that human intellectual and critical content to tease out the real meaning to us as designers and therefore become something slightly different.”
Input determines outcome
Given the dependence of AI output on the data that are input for training, another consideration for Tsigkari is the quality of AEC datasets. “There is one universal truth behind AI: data is king,” she said. “If we want to use and control these technologies to the best of our ability, we need to learn to control the data that drives them first.”
She noted the need for consistent tagged building datasets that are “contextualized, socially appropriate, structurally viable, sustainability sensitive and code complying. Our first challenge is to collect, organize and process our data across disciplines in a meaningful manner so that we can leverage it. Deploying in-house trained—rather than pre-trained—models is also a very robust way of ensuring the quality of your results,” she added.
Creativity balances AI and CHI
As AI becomes more embedded in the work of architecture, how does the definition of creativity change? Tang evoked the “Star Trek” character Data when discussing the imperative of human agency to refine the outcomes AI generates. “Data is an artificial intelligent being constantly looking for the human side of himself,” Tang explained. “I don’t think AI can ever supersede or replace human intelligence, particularly CHI.”
Tsigkari noted that humans have the upper hand on several qualities that enable creativity—including aesthetics, emotion, collaboration, communication and responsibility. “We should be focusing on how AI can become a creative assistant that is augmenting, rather than replacing, creativity—and the values we bring to the table are driving the changes we want to see.”